Navigating the Waves of Market Sentiment: The Role of NLP in Stock Analysis
In the ever-shifting landscape of the financial markets, understanding investor sentiment has become a vital compass for navigating the tides of stock performance. As traditional analytical methods often fall short in capturing the nuances of human emotion and market psychology, a new ally has emerged: Natural Language Processing (NLP). This innovative technology, steeped in artificial intelligence, is revolutionizing the way traders interpret the vast oceans of data generated by news articles, social media posts, and financial reports. By harnessing the power of language and context, NLP tools are transforming raw text into actionable insights, offering a lens through which market sentiment can be quantified and analyzed. In this article, we will explore how NLP is reshaping stock market analysis, providing an avenue for investors to glimpse beyond the numbers, while uncovering the underlying emotions that drive market movements. Join us as we delve into the synergy of linguistics and finance, and discover how sentiment analysis is carving a new path in the world of investing.
Table of Contents
- Understanding Market Sentiment through Natural Language Processing
- Techniques for Analyzing Social Media and Financial News
- Building a Sentiment Analysis Model for Stock Predictions
- Practical Applications and Ethical Considerations in NLP for Finance
- Q&A
- Concluding Remarks
Understanding Market Sentiment through Natural Language Processing
In the realm of finance, understanding the prevailing mood of investors is crucial for making informed trading decisions. Natural Language Processing (NLP) plays a pivotal role in analyzing vast amounts of unstructured data, such as news articles, social media posts, and earnings reports. By leveraging *sentiment analysis* techniques, businesses can decode the emotional tone behind these texts, categorizing them as positive, negative, or neutral. This information offers valuable insights into market trends and investor behavior, providing a deeper understanding of the factors influencing stock prices. Key elements that NLP focuses on include:
- Public Sentiment: Gauging the overall sentiment towards specific stocks or the market itself.
- Emotion Detection: Identifying various emotions such as fear, excitement, or optimism prevalent in investor communications.
- Trend Analysis: Obs
erving shifts in sentiment over time to predict future market movements
By applying sophisticated algorithms to financial texts, NLP can offer actionable insights that were previously difficult to attain. Furthermore, predictive modeling can help investors anticipate the potential impact of news events on stock performance. For instance, a sudden surge of negative sentiment following a major announcement can signal a downturn, while a positive buzz surrounding earnings could indicate bullish trends. To illustrate the advantages of using NLP in this context, consider the table below showcasing sentiment scores for a selection of recent articles:
Article Title | Sentiment Score | Market Impact Prediction |
---|---|---|
Company A’s Stellar Q3 Earnings | +0.85 | Positive |
Company B Faces Regulatory Hurdles | -0.78 | Negative |
Market Reactions to Global Economic Policies | +0.02 | Neutral |
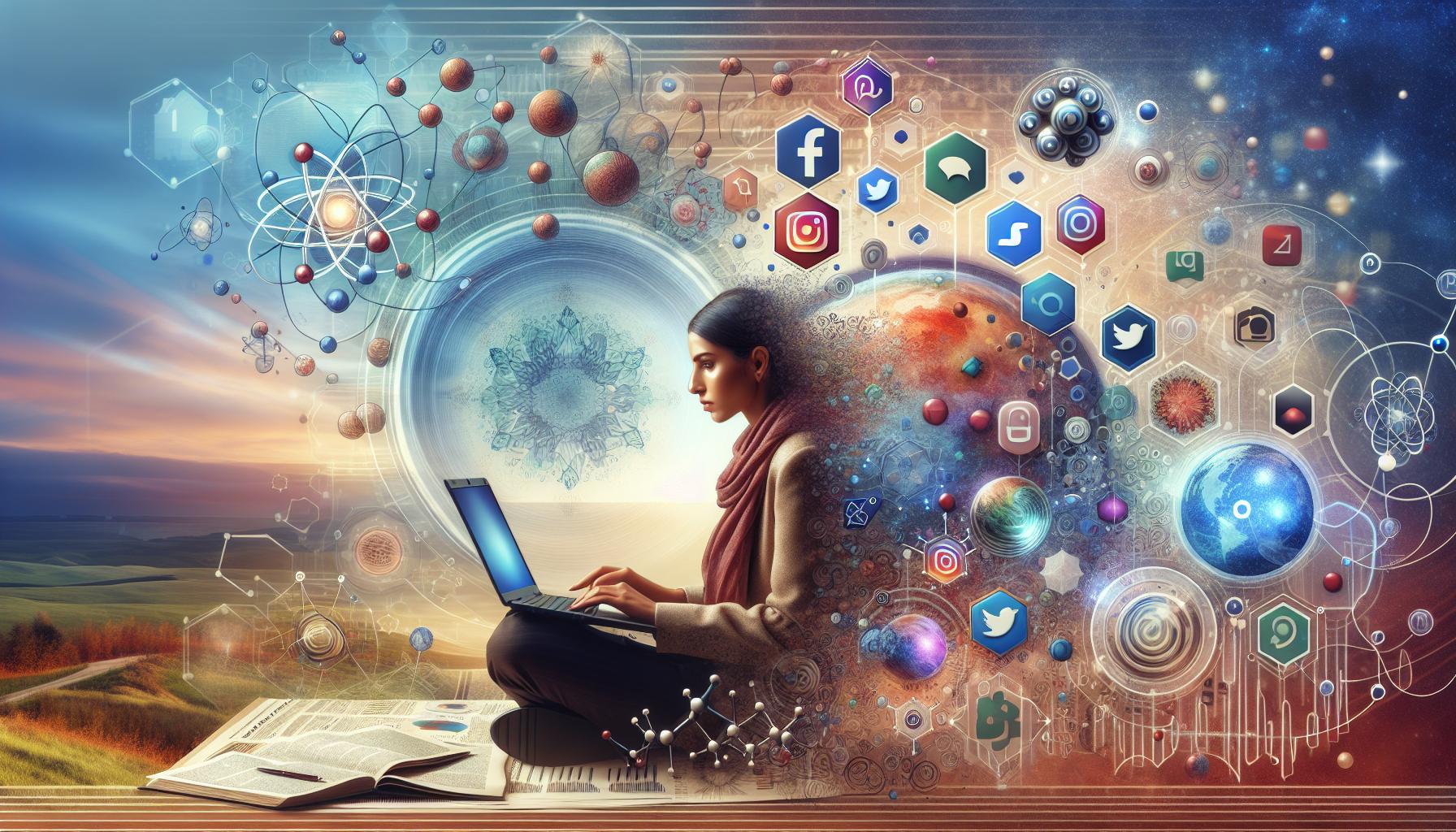
Techniques for Analyzing Social Media and Financial News
To effectively analyze sentiment from social media and financial news, researchers often employ various Natural Language Processing (NLP) techniques. These methods allow for the dissection and interpretation of vast amounts of textual data to determine overall market sentiment. Some of the primary techniques used include:
- Sentiment Analysis: This involves classifying text as positive, negative, or neutral based on the words and phrases used.
- Topic Modeling: By identifying recurring themes within texts, this technique helps in understanding what specific issues are driving public sentiment.
- Named Entity Recognition (NER): Extracting names of companies, products, and other relevant entities provides insight into which subjects are trending in discussions.
- Time-Series Analysis: Integrating sentiment scores with stock price movements over time offers a clearer picture of sentiment’s impact on market behavior.
For a comprehensive analysis, it’s crucial to use a combination of these techniques, often enhanced by machine learning algorithms that allow for predictive modeling. To illustrate the impact of sentiment on stock prices, consider the following table that correlates sentiment scores from Twitter with corresponding stock movements:
Time Period | Avg. Sentiment Score | Stock Price Movement (%) |
---|---|---|
Week 1 | 0.45 | +3.5% |
Week 2 | -0.20 | -2.1% |
Week 3 | 0.60 | +4.8% |
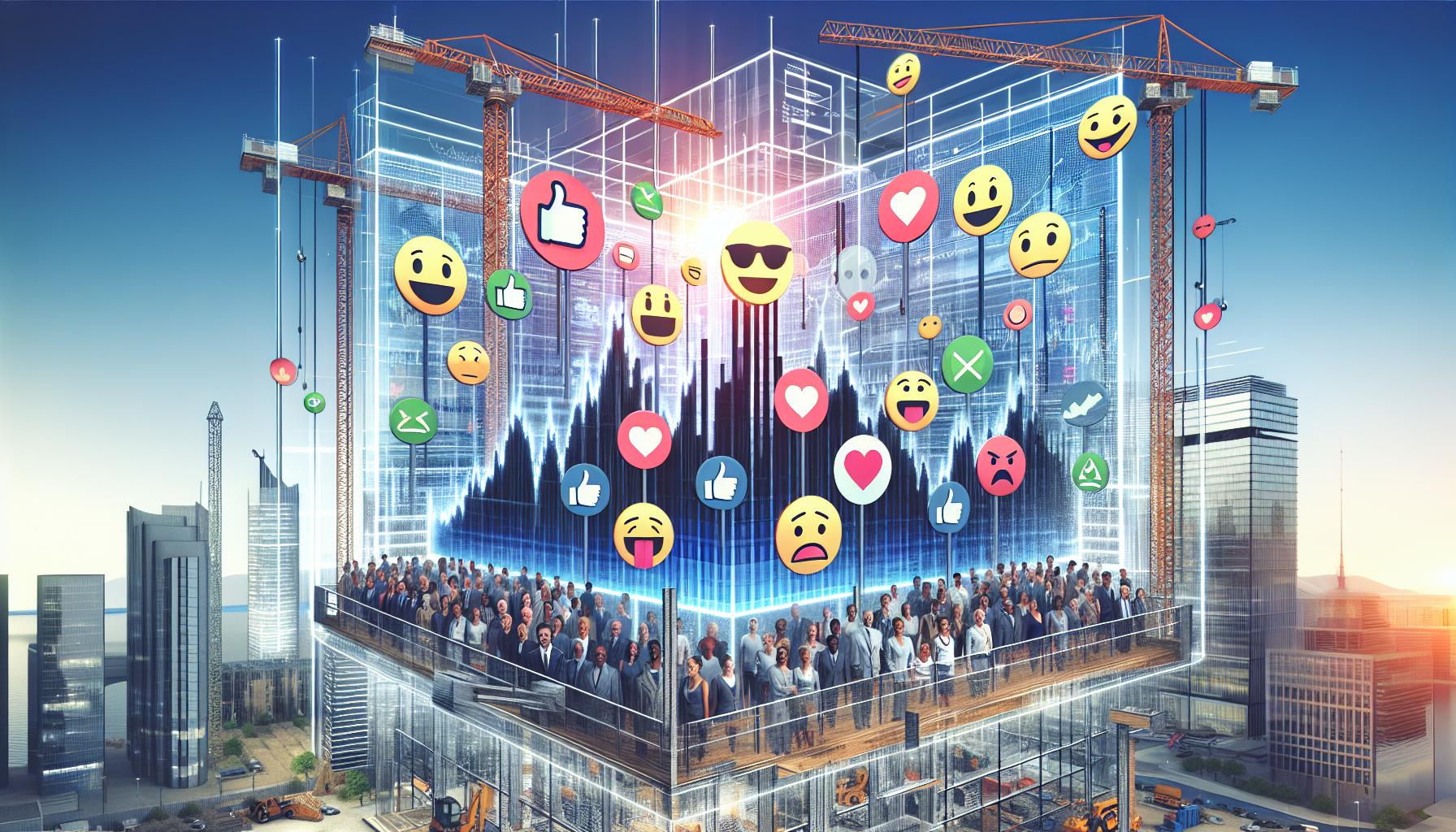
Building a Sentiment Analysis Model for Stock Predictions
Creating an effective sentiment analysis model for stock predictions involves harnessing the power of Natural Language Processing (NLP) to glean insights from various textual sources. By analyzing sentiments reflected in news articles, social media interactions, and analysts’ reports, one can uncover underlying trends that traditional financial indicators might overlook. Key components to consider while building this model include:
- Data Collection: Gather diverse datasets from platforms like Twitter, financial news sites, and stock market forums.
- Text Preprocessing: Cleanse the data by removing noise—such as stop words, punctuation, and irrelevant content—to enhance model accuracy.
- Sentiment Labeling: Utilize techniques like manual tagging or automated tools to classify sentiments as positive, negative, or neutral.
Once the initial groundwork is laid, the next steps involve employing machine learning techniques to train the model. This can be achieved through various algorithms ranging from simple logistic regression to more complex neural networks. To optimize predictions, consider using:
- Feature Extraction: Explore methods like TF-IDF or word embeddings to transform text data into numerical features.
- Model Evaluation: Implement metrics such as precision, recall, and F1-score to assess the model’s performance.
- Backtesting: Validate model predictions against historical stock prices to familiarize with its efficacy in real-world trading scenarios.
Key Indicator | Relevance to Model |
---|---|
Market Sentiment | Directly affects stock prices, guiding investors’ decisions. |
Public Opinion | Shapes market trends and influences stock volatility. |
Brand Reputation | Impacts company performance and stock stability. |
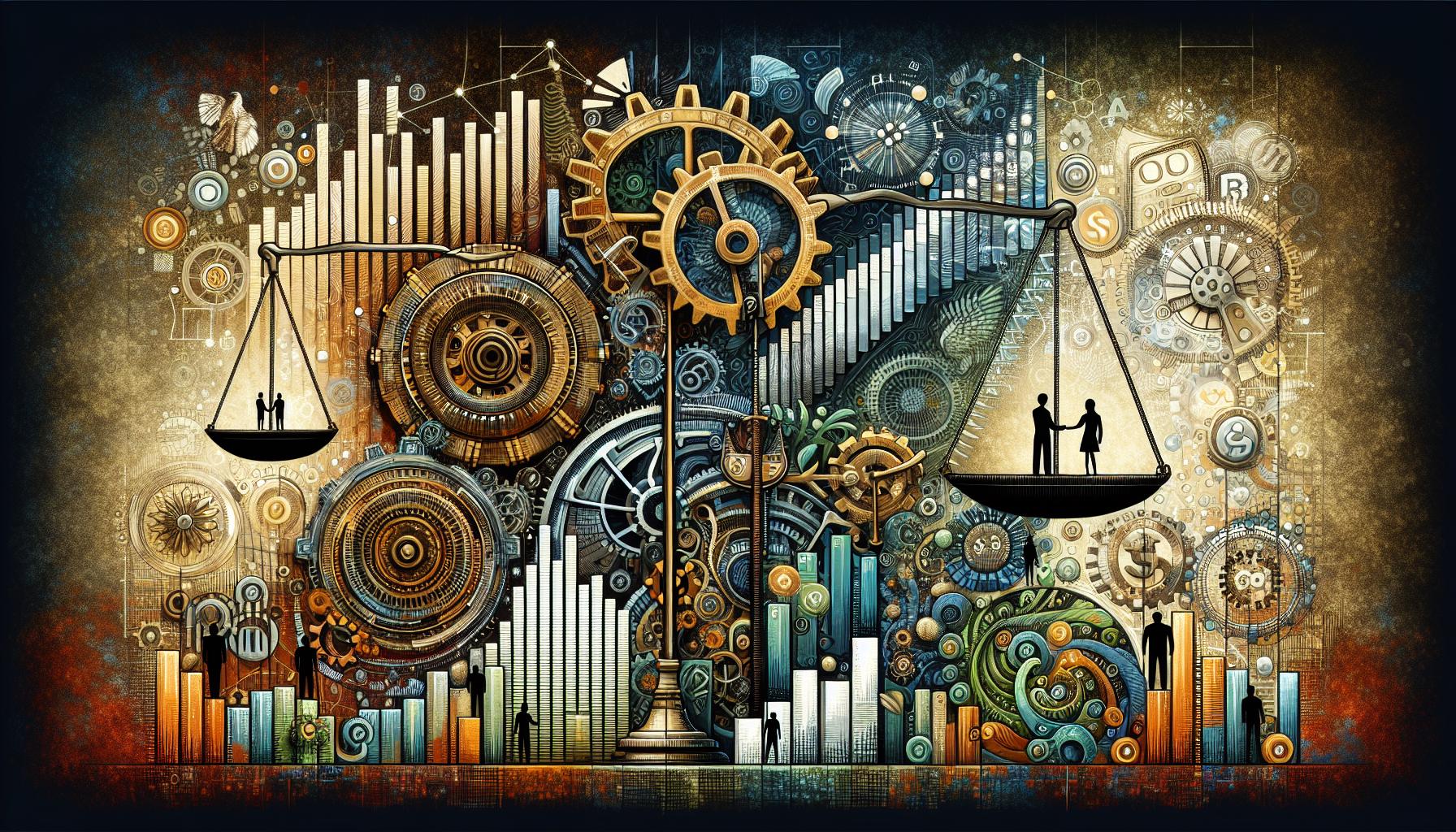
Practical Applications and Ethical Considerations in NLP for Finance
Natural Language Processing (NLP) has opened new avenues in finance, particularly in stock market sentiment analysis. By harnessing vast amounts of textual data from news articles, social media, and financial reports, financial analysts can glean insights that inform trading strategies. Key applications include:
- Sentiment scoring: Assessing public sentiment regarding specific stocks or market trends.
- Market prediction: Anticipating stock price movements based on sentiment data derived from NLP.
- Risk assessment: Evaluating potential risks by analyzing the negativity or positivity in news content.
NLP tools empower traders to react swiftly to changes in market sentiment, potentially leading to smarter investment decisions.
However, the use of NLP in finance isn’t without its ethical dilemmas. The reliance on algorithms raises concerns regarding data privacy and the potential misuse of sensitive information. Crucial ethical considerations encompass:
- Data consent: Ensuring that publicly available data sources respect individual privacy and consent.
- Bias and fairness: Addressing inherent biases in NLP algorithms that could skew analysis or misrepresent markets.
- Market manipulation: Safeguarding against the misuse of sentiment analysis for malicious purposes.
Establishing stringent regulations and ethical guidelines is imperative to maintain market integrity while leveraging the transformative potential of NLP technologies.
Q&A
Q&A: NLP for Stock Market Sentiment
Q1: What is NLP, and how does it relate to stock market sentiment?
A: NLP, or Natural Language Processing, is a branch of artificial intelligence that focuses on the interaction between computers and human language. In the context of the stock market, NLP analyzes news articles, social media posts, earnings reports, and more to gauge the sentiment surrounding a particular stock or the market as a whole. By processing vast amounts of textual data, NLP tools can identify whether the overall sentiment is positive, negative, or neutral, which can influence investment decisions.
Q2: Why is sentiment analysis important for investors?
A: Sentiment analysis can provide insights into market trends and public perception, which are often driving factors for stock prices. Understanding general sentiment can help investors anticipate price movements, as market reactions frequently align with prevailing emotions and opinions. For instance, positive sentiment around a company’s earnings announcement may indicate a potential rise in stock value, while negative sentiment may suggest the opposite.
Q3: What types of data can NLP analyze for stock market sentiment?
A: NLP can analyze a wide range of textual data, including but not limited to financial news articles, social media platforms (like Twitter, Reddit, and StockTwits), earnings call transcripts, analyst reports, and even financial blogs. This diverse set of data sources enables NLP models to capture a holistic view of the sentiment surrounding a stock, taking into account various perspectives and narratives.
Q4: How does NLP determine whether sentiment is positive or negative?
A: NLP algorithms typically use a combination of techniques, such as keyword extraction, sentiment lexicons, and machine learning models, to classify sentiment. For example, they might analyze the presence of positive or negative words (like “growth” vs. “loss”) and assess context to understand sentiment nuances. Advanced models, especially those based on deep learning, can even recognize sarcasm or mixed sentiments by analyzing patterns in language use.
Q5: What are the challenges of using NLP for stock market sentiment analysis?
A: One of the primary challenges is the dynamic and often unpredictable nature of financial markets. Sentiment is influenced by numerous factors, including global events, economic data, and investor psychology, which can be difficult for algorithms to quantify. Additionally, ambiguous language and context-specific phrases can lead to misinterpretation. Continuous refinement of models and access to high-quality, relevant data are essential for improving accuracy.
Q6: How can NLP be integrated into an investor’s strategy?
A: Investors can use NLP-driven sentiment analysis as one tool among many in their decision-making arsenal. By incorporating sentiment data into their analyses—complementing traditional metrics like financial ratios, technical indicators, and market trends—they can enhance their understanding of market movements. Furthermore, automated trading systems can utilize sentiment scores to inform buy or sell signals, potentially providing a competitive edge.
Q7: Are there any particular tools or platforms that leverage NLP for stock market sentiment?
A: Yes, numerous tools and platforms have emerged that utilize NLP for sentiment analysis. Examples include Bloomberg Terminal, RavenPack, and Accern, which offer specialized analytics and insights for traders. Additionally, open-source libraries like NLTK, spaCy, and Hugging Face’s Transformers allow tech-savvy investors to build their own sentiment analysis models tailored to specific stocks or sectors.
Q8: What does the future hold for NLP in stock market sentiment analysis?
A: The future looks promising as advancements in NLP technology continue to evolve. With the integration of more sophisticated algorithms and increased computational power, we can expect improved accuracy and real-time analysis capabilities. Moreover, as behavioral finance highlights the importance of psychology in investing, sentiment analysis will likely become an even more critical component of comprehensive investment strategies in the years to come.
Q9: Can NLP truly predict stock market movements?
A: While NLP can provide valuable insights into market sentiment and potential future trends, it’s essential to remember that predicting stock market movements with absolute certainty remains a challenge. The markets are influenced by numerous unpredictable factors, including economic data, geopolitical events, and sudden shifts in investor behavior. Therefore, while NLP is a powerful tool that can enhance decision-making, it should not be relied upon in isolation for investment decisions.
Concluding Remarks
As we navigate the ever-shifting tides of the stock market, the integration of Natural Language Processing offers a beacon of clarity amid the noise. By harnessing the nuances of language and sentiment, NLP empowers investors and analysts to make informed decisions based on real-time insights. As technology continues to evolve, so too will our ability to interpret and act upon the collective emotions of the market—turning data into discernible patterns, and predictions into opportunities.
In embracing these innovations, we stand at the forefront of a new era in investing, where understanding sentiment can be as crucial as evaluating stock fundamentals. Looking ahead, the synergy between human intuition and machine intelligence holds limitless potential. As we deepen our understanding of market psychology, we pave the way for more nuanced investment strategies and a richer comprehension of economic trends. In this dynamic interplay of technology and finance, the quest for insight remains limitless, promising a future where informed decision-making thrives in the face of uncertainty.